Measuring health: How we use (and sometimes don’t use) DALY estimates
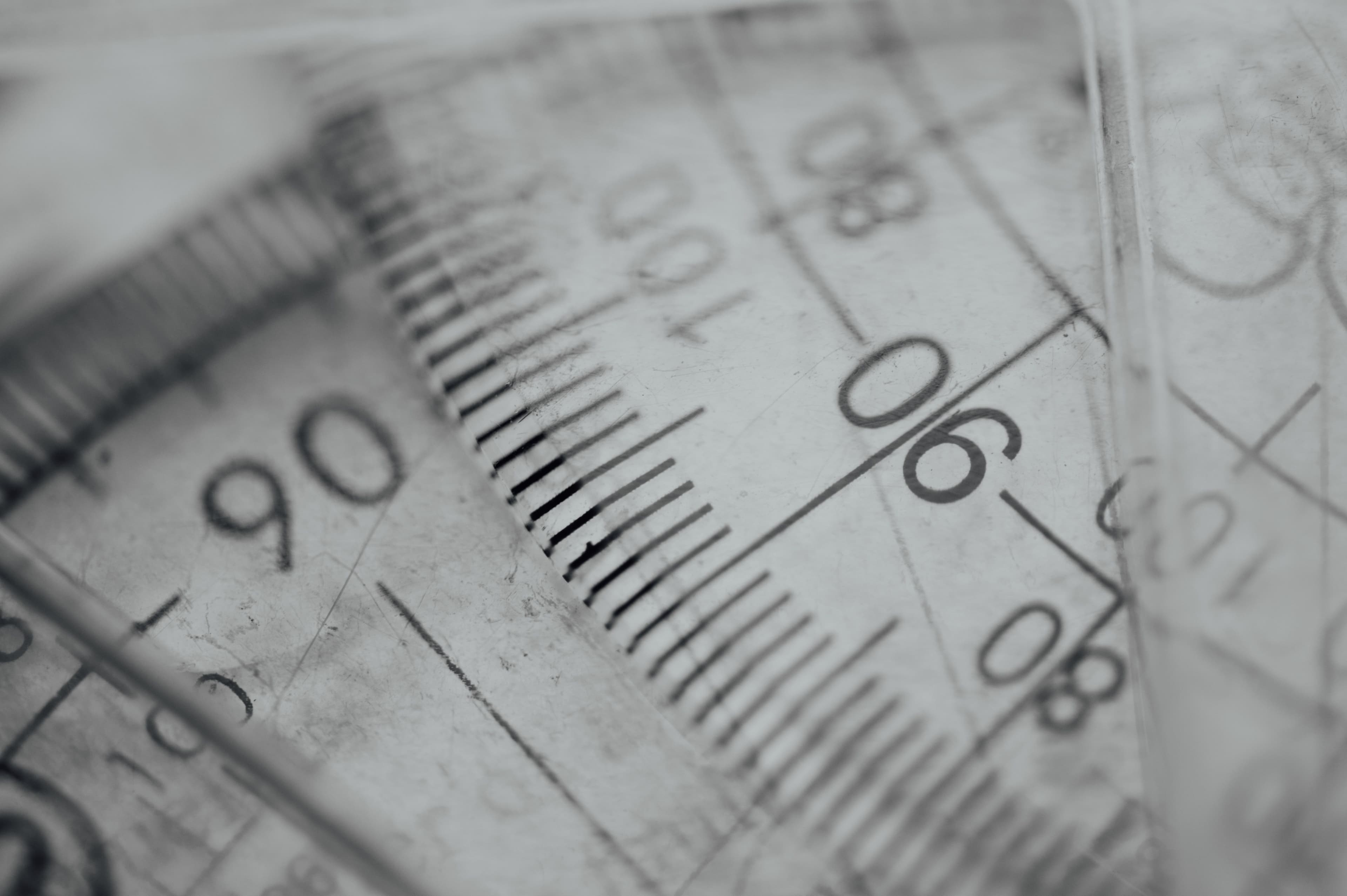
▲ Photo by CHUTTERSNAP on Unsplash
Disability-Adjusted Life Years (DALYs) are one of the most well-used metrics within global health, and are the only metric that quantifies the burden of diseases across countries with a high level of coverage. However, they also present several limitations for conducting charity evaluation and cause area exploration. This post lays out how we think about and use DALYs, which involves accounting for these limitations and often using other metrics where possible. Overall, we think that this approach maximizes our ability to identify impactful ways to do good in the world.
At Founders Pledge, we aim to find the most effective ways to do good through charitable giving. This means that we need a yardstick by which to contrast the impact of the different funding opportunities that we are investigating. Within the area of global health, we want to be able to capture the degree to which a charity improves health and wellbeing. Having such a measure makes it possible to compare different charities, and also gives us a starting point from which to identify new cause areas. For example: which diseases have the greatest deleterious impact, and in which geographic areas?
One of the most frequently used metrics within global health is the Disability-Adjusted Life Year (DALY). This WHO-endorsed metric attempts to capture the overall burden of different diseases, and includes both the effect of that disease upon mortality and the burden it imposes by causing illness and disability. These DALY estimates are produced by the Global Burden of Disease study, a huge collaborative research program led by the Institute of Health Metrics and Evaluation at the University of Washington and involving over 7000 researchers from around the world.1 A significant advantage of DALYs is that they have been estimated for a wide array of conditions, in a large number of places; DALY estimates cover 369 different diseases and injuries, for 204 countries and territories.2 At present, there is no other comparable metric with this kind of coverage.
Overall, our goal is to compare the ability of different interventions and programs to do good in the world. DALYs estimate the health impact of a given disease, and are therefore a helpful tool to pinpoint key causes of suffering. However, they cannot provide all the information that we need—improving health is only one facet of doing good work, and some interventions make their impact through other pathways. For example, some charities work to improve people’s economic circumstances, or their mental wellbeing. In addition, DALYs are measured using data about people’s subjective judgements (essentially, whether they think disease A or disease B is worse), but we know that people’s expectations about how bad a given disease is do not necessarily agree with the lived experience of people who actually suffer from it. Consequently, DALYs need to be interpreted in context in order to ensure the highest-quality evidence-led philanthropy. The purpose of this post is to lay out our approach to using DALYs, within cause area exploration and charity evaluation.
What is a DALY?
The cost of a disease in DALYs is an estimation of that disease’s overall health burden—including its effect upon mortality, as well as the burden it imposes by causing illness and disability. It is the sum of two measures: (1) the years of life lost as a result of that condition and (2) the years of life lived with disability. For example, a DALY estimate for the burden of malaria in India would be as follows:
DALY (malaria in India) = YLL (malaria in India) + YLD ( malaria in India)
Of the two measures, the Years of Life Lost (YLL) is more straightforward; this is the estimated number of years of life lost as a consequence of this disease. It is the number of cause-specific deaths (here, the number of deaths due to malaria in India) multiplied by the average years of life lost due to that disease—so a disease that causes mortality early in life will generate a higher YLL estimate.3
The Years Lost due to Disability (YLD) is more complex. YLD attempts to capture the health-related impact of living with the disease (beyond mortality). In our example, this is the estimate of the health impacts of living with malaria—the burden of suffering from symptoms such as fever, nausea and so on. The severity of living with these symptoms is captured by the disability weight, which is multiplied by the disease’s prevalence;
YLD (malaria in India) = disability weight (malaria in India) x prevalence (malaria in India)
Taken together, DALY estimates therefore provide a global metric for contrasting the burdens of different diseases across geographic regions, including impacts on mortality as well as on illness and disability. All the information that is captured within a DALY estimate—including the years of life lost, prevalence, and the disability weight of a given condition—is captured by consolidating data from numerous different sources, such as national vital registration data and estimates from WHO technical programs, and by commissioning huge surveys within different countries. This data is then processed by scientists and WHO affiliates,4 and is available here.
Problems with disability weights
We use DALY estimates to help us identify promising cause areas (for example, by asking which diseases carry a high DALY burden, yet receive relatively little funding), and sometimes to help us estimate the cost-effectiveness of a given charity. However, we also recognize their limitations in these areas—in particular, we are often skeptical of the methods used to generate the disability weights.
Disability weights are constructed by surveying thousands of people in the general population and asking whether they think that person A (who has a particular condition) is more or less healthy than person B (who has a different condition). Survey respondents are provided with a short description of each condition’s primary consequences and symptoms, making it easier for the average person to assess the severity of that condition. See the example below, in which Person A is suffering from dementia, while person B is suffering from diabetic neuropathy, a form of nerve damage that can occur in people with diabetes.5
Person A has memory problems and confusion, feels disoriented, at times hears voices that are not real, and needs help with some daily activities.
Person B has pain, tingling and numbness in the arms, legs, hands and feet. The person sometimes gets cramps and muscle weakness.
Do you think that person A, or person B, is healthier overall?
Each survey respondent receives ~15 of these pairwise comparisons, covering different pairs of diseases; the pairs are drawn at random from the entire sample of diseases.6 The resulting data is then ordered, using mathematical techniques based on the proportion of the time that each condition is ranked as being more or less healthy across these pairwise comparisons. For example, if a person suffering from dementia is generally ranked as being less healthy than people suffering from other conditions, this will push dementia towards a higher ranking (meaning that its disability weight is estimated to be more severe).
These surveys have been undertaken in-person (for example, in Tanzania, Bangladesh, Indonesia and Peru), over the phone (in the US) and over the internet.7 Importantly for the credibility of the methodology, the results of these surveys appear to be fairly consistent across countries.8 The results of these surveys generate the disability weights, which are consistent across countries and available here.
Estimating wellbeing impact
A key point is that disability weights aim to capture the health impact of a given disease—but we are typically aiming to understand how a given disease negatively impacts upon people’s happiness. At first glance, it seems reasonable to assume that the health impact of a given disease will correlate well with its impact upon wellbeing. But actually, the relationship seems to be quite complex—for example, diseases such as mental health disorders have a relatively small disability weight, but may have a disproportionately large impact upon wellbeing; see Fig 1.
Fig 1: Average decreases in life satisfaction points (on a scale of 0 - 10), versus disability weightings of particular diseases. The data on life satisfaction points is taken from analyses of European adults,9 while the disability weightings come from the Global Burden of Disease study.10 Note that this data only covers a relatively small subset of diseases—however, it does suggest that mental health disorders could have an especially strong impact upon life satisfaction relative to their disability weight.
To mitigate this problem, we use measures that directly assess wellbeing (rather than DALYs), where possible. For example, we can calculate the impact of a given charity in terms of WELLBYs (Wellbeing-Adjusted Life Years): one less WELLBY is a loss in life satisfaction of 1 point on a 1-10 scale, for one year.11 At present, wellbeing data has only been estimated for a small subset of conditions, and only with a very limited sample. Some of the charities that we evaluate also operate by improving people’s economic circumstances, or by directly reducing mortality—allowing us to calculate their impact in terms of economic or mortality metrics rather than using DALYs.
Nonetheless, there are some circumstances where it is necessary to use DALY estimates to understand a charity’s impact. This means that we need to work out how our DALY estimates translate into effects upon wellbeing; can we trust a DALY estimate for the impact of depression to the same extent that we trust an estimate for the impact of malaria?
Going forward, we will continue to use DALYs to measure health improvements— but we will also integrate other ways of measuring quality-of-life. We are able to do this by taking advantage of other wellbeing effect estimates that have been produced—such as the wellbeing effects of cash transfers,12 estimates of the value of a life compared to cash transfer value,13 and how people value a year of life lost (which we can convert to a DALY estimate). This allows us to convert between different measurements, using the most relevant metric for estimating the impact of a given condition.
Are disability weights universal?
Disability weight data is constructed by asking people to judge the severity of conditions against each other. However, this means that people are judging the severity of diseases which they themselves have not necessarily suffered from—and some diseases may have a heavier burden in particular cultural contexts.
Ever since early estimates were released, there have been concerns about whether disability weights can reasonably be considered universal. Critics have argued that it may be impossible to separate a health status from sociocultural context when estimating severity.14 For example, the severity of blindness might be greater in a lower income country relative to a higher income country which has greater access to assistive technology.15.
While a number of surveys took place in low and middle-income countries, it is notable that the majority of people who undertake the surveys to measure disability weight were in Europe and completed the surveys over the internet.16 The extent to which this is a problem is unclear. The authors of these studies have argued that rankings are similar across countries and surveying methods,17 but it could be the case that the impact of conditions that people in high income countries do not suffer from, or will likely only experience a milder form of, is underestimated. This is a particular issue for us, since most of the charities we recommend work in low-income countries.
This problem came up in our research work looking at oral health disorders; while most people in high income countries have experienced a cavity, they have not experienced the more severe forms of oral disorder (such as a dental abscess). We think this makes it likely that the severity of oral health disorders has been underestimated with respect to regions with poor access to dental treatment, and is therefore underweighted in DALY estimates.
In practice, it is difficult to understand the extent of the universality problem (and therefore to adequately correct for it) without more detailed analyses or datasets which examine regional differences in global disability weightings. In the meantime, we examine on a case-by-case basis whether particular diseases are liable to be underestimated via DALY weightings as we proceed with charity comparisons—and convert to wellbeing measures where possible.
Are disability weights skewed by cognitive biases?
It is well-established that human judgment is not perfectly rational—we exhibit a number of systematic patterns of deviation from perfectly rational thinking, known as cognitive biases.18 We should expect to see these biases when we examine disability weight data.
In particular, it has been argued that the ‘focusing illusion’ skews our predictions of disease severity.19 The focusing illusion is the tendency to focus narrowly on attention grabbing aspects of a particular disease. For example, in considering the severity of life with a colostomy bag, we might zone in on images of plastic pouches, or the difficulty of wearing a swimming costume. However, we fail to consider the hundreds of daily behaviors that would be unchanged.20 Applied to the contexts of disability weights, this means that people may overstate the impact of diseases with dramatic symptoms, and underestimate the impact of diseases which are less ‘attention-grabbing’—such as the impact of chronic pain or loneliness.21
In addition, people often fail to account for hedonic adaptation—the tendency for people to return to a ‘baseline’ level of happiness following either a positive or negative life event.22 For example, we predict that we will become far happier if we win the lottery, but real-life evidence from lottery winners suggests that they return to their baseline level of happiness surprisingly quickly.23 It has been suggested that people also fail to account for hedonic adaptation when predicting disease severity; that is, we forget that people tend to adjust to new health circumstances.24 This mechanism might explain the disability paradox, or the finding that patients with chronic disabilities tend to report that their quality of life is significantly better than the public estimates that it will be.25 However, certain diseases (such as mental illness and chronic pain conditions) are thought to be especially difficult to adjust to. This suggests that the impact of illnesses which are less subject to hedonic adaptation are underestimated, relative to illnesses which people are better able to adjust to.26
Overall, we should not expect that people’s predictions about ‘which person is healthier’ will necessarily map onto people’s lived experience of a given disease. For this reason, we aim to incorporate different data sources (for example, data which directly links living with a given disease to its impact upon wellbeing) where possible.
Conclusions
DALYs have enabled philanthropists and global health experts to better quantify global health impacts, and are a hugely useful measure in many contexts. In addition, this data is freely available to everyone, and often gives us a place to start. However within the context of cause area identification and charity evaluation, DALYs also present a number of drawbacks. In particular, DALYs aim to measure health impacts—but there are many facets beyond physical health that determine someone’s experience of the world. Consequently, over-reliance upon DALYs risks missing interventions that act through other means to create positive impact. We are also skeptical about the degree to which disability weights truly capture the real-world impacts of suffering from many diseases; for this reason, we prioritize using direct measurements of wellbeing where possible. Overall, accounting for the limitations of DALYs—without neglecting their use completely—maximizes our ability to identify the most impactful ways to do good in the world.
Notes
-
‘About GBD’, Institute for Health Metrics and Evaluation, 18 April 2014, https://www.healthdata.org/gbd/about. ↩
-
‘GBD History’, Institute for Health Metrics and Evaluation, 18 April 2014, https://www.healthdata.org/gbd/about/history. ↩
-
WHO, ‘WHO Methods and Data Sources for Global Burden of Disease Estimates 2000-2019’, December 2020, https://cdn.who.int/media/docs/default-source/gho-documents/global-health-estimates/ghe2019_daly-methods.pdf?sfvrsn=31b25009_7. ↩
-
‘Global Health Estimates’, accessed 8 August 2022, https://www.who.int/data/global-health-estimates; Joshua A Salomon et al., ‘Common Values in Assessing Health Outcomes from Disease and Injury: Disability Weights Measurement Study for the Global Burden of Disease Study 2010’, The Lancet 380, no. 9859 (2012): 2129–43; ‘GBD History’. ↩
-
Joshua A Salomon et al., ‘Disability Weights for the Global Burden of Disease 2013 Study’, The Lancet Global Health 3, no. 11 (2015): e712–23. ↩
-
Juanita A. Haagsma et al., ‘Assessing Disability Weights Based on the Responses of 30,660 People from Four European Countries’, Population Health Metrics 13, no. 1 (3 April 2015): 10, https://doi.org/10.1186/s12963-015-0042-4. ↩
-
Joshua A Salomon et al., ‘Disability Weights for the Global Burden of Disease 2013 Study’, The Lancet Global Health 3, no. 11 (2015): e712–23. ↩
-
Salomon et al., ‘Common Values in Assessing Health Outcomes from Disease and Injury: Disability Weights Measurement Study for the Global Burden of Disease Study 2010’, 2012. ↩
-
‘WALY | Happiness Research Institute’, happiness research, accessed 30 May 2022, https://www.happinessresearchinstitute.com/waly. ↩
-
‘GBD Results Tool | GHDx (2019)’, accessed 21 March 2022, https://ghdx.healthdata.org/gbd-results-tool. ↩
-
Michael Plant, ‘The Measurement of Wellbeing’, Happier Lives Institute, accessed 8 August 2022, https://www.happierlivesinstitute.org/report/the-measurement-of-wellbeing. ↩
-
Joel McGuire, Caspar Kaiser, and Anders Bach-Mortensen, ‘The Impact of Cash Transfers on Subjective Well-Being and Mental Health in Low-and Middle-Income Countries: A Systematic Review and Meta-Analysis’, 2020. ↩
-
‘Measuring People’s Preferences and Values to Inform Funding Decisions’, accessed 10 August 2022, https://www.idinsight.org/project/using-peoples-preferences-and-values-to-inform-funding-decisions. ↩
-
‘Opening the Debate on DALYs | Health Policy and Planning | Oxford Academic’, accessed 8 August 2022, https://academic.oup.com/heapol/article/11/2/179/656807; Ariel Chen et al., ‘The Evolution of the Disability-Adjusted Life Year (DALY)’, Socio-Economic Planning Sciences 49 (2015): 10–15; Erik Nord, ‘Uncertainties about Disability Weights for the Global Burden of Disease Study’, The Lancet Global Health 3, no. 11 (1 November 2015): e661–62, https://doi.org/10.1016/S2214-109X(15)00189-8. ↩
-
Chen et al., ‘The Evolution of the Disability-Adjusted Life Year (DALY)’. ↩
-
It is possible that statistical measures were taken to proportionately weight the responses of people from different countries/ economic groups according to their global representation; I struggled to find much information about this, and could not find evidence for this in the documents I read from the WHO about their methods (e.g. WHO, 2020). I would welcome any further information about this. ↩
-
Joshua A Salomon et al., ‘Common Values in Assessing Health Outcomes from Disease and Injury: Disability Weights Measurement Study for the Global Burden of Disease Study 2010’, The Lancet 380, no. 9859 (2012): 2129–43. ↩
-
Daniel Kahneman and Amos Tversky, ‘On the Reality of Cognitive Illusions.’, 1996. ↩
-
Peter A Ubel et al., ‘Misimagining the Unimaginable: The Disability Paradox and Health Care Decision Making.’, Health Psychology 24, no. 4S (2005): S57; ‘WALY | Happiness Research Institute’. ↩
-
Ubel et al., ‘Misimagining the Unimaginable: The Disability Paradox and Health Care Decision Making.’ ↩
-
Daniel Kahneman et al., ‘Would You Be Happier If You Were Richer? A Focusing Illusion’, Science 312, no. 5782 (2006): 1908–10; David A Schkade and Daniel Kahneman, ‘Does Living in California Make People Happy? A Focusing Illusion in Judgments of Life Satisfaction’, Psychological Science 9, no. 5 (1998): 340–46. ↩
-
Sonja Lyubomirsky, Hedonic Adaptation to Positive and Negative Experiences. (Oxford University Press, 2011). ↩
-
Philip Brickman, Dan Coates, and Ronnie Janoff-Bulman, ‘Lottery Winners and Accident Victims: Is Happiness Relative?’, Journal of Personality and Social Psychology 36, no. 8 (1978): 917. ↩
-
‘WALY | Happiness Research Institute’; Ubel et al., ‘Misimagining the Unimaginable: The Disability Paradox and Health Care Decision Making.’ ↩
-
Gary L Albrecht and Patrick J Devlieger, ‘The Disability Paradox: High Quality of Life against All Odds’, Social Science & Medicine 48, no. 8 (1999): 977–88. ↩
-
Heli Koivumaa-Honkanen et al., ‘Life Satisfaction and Depression in a 15-Year Follow-up of Healthy Adults’, Social Psychiatry and Psychiatric Epidemiology 39, no. 12 (2004): 994–99. ↩