How we think about charity evaluation
How we conduct charity evaluation, and the conceptual tools and models we use in the research process.
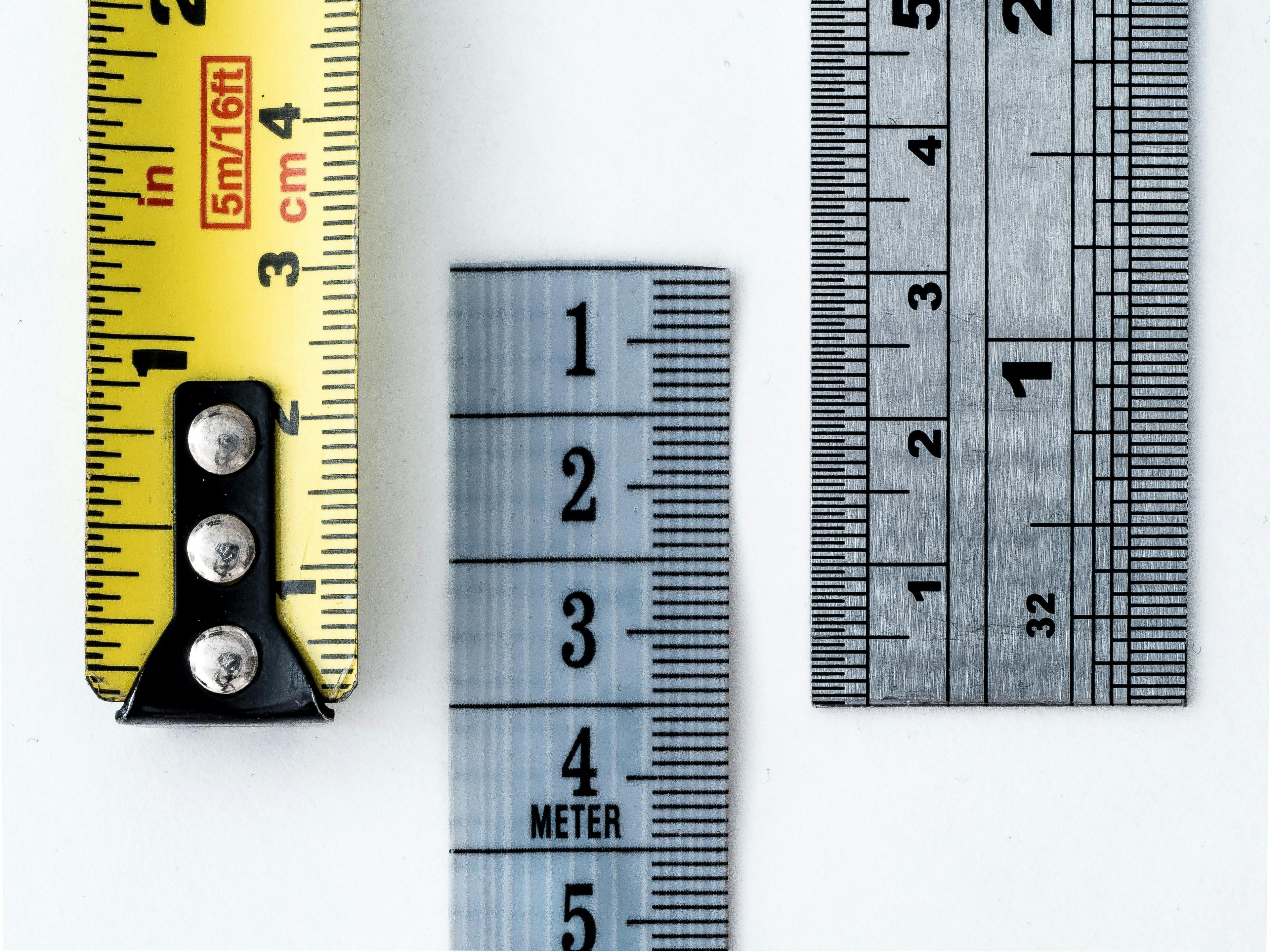
▲ Photo by William Warby on Unsplash.
Related article
Why charity evaluation matters
There are many reasons people give to charity. They give to support an emotionally resonant cause, to fulfill an obligation to their communities, or to “pay forward” their own good fortune. At Founders Pledge, we’re concerned with one motivation above all others: to make the largest possible contribution to making a better world. Our approach is completely focused on the good we can do, and completely impartial about who it’s done for.
We think there is broad agreement on what a better world looks like. A better world is one in which fewer people die young, in which all conscious creatures are valued and protected, in which more people are happier, freer, and more prosperous, and in which there is less disease, trauma, and pain. The world we imagine is sustainably better; in fact, it is improving every day.
The world we live in now is dauntingly far from this goal. It’s not just that people live in conditions of severe deprivation, that they lose children to malaria or suffer from crippling depression. There’s also the possibility that the world itself could cease to exist as we know it. As history advances, humans increasingly have the means to inflict pain and death on a mass scale: we could destroy ourselves as a side effect of a big war or by accident with a man-made plague; advanced technology could enable bad actors to someday impose a permanent authoritarianism from which there is no chance of escape.
These are difficult challenges, but not insurmountable ones. In the past, philanthropic initiatives have funded research that saved a billion lives, caused dramatic reductions in nuclear arsenals, and reduced the incidence of malaria in sub-Saharan Africa by 40%. In recent years, evidence-minded donors have started to focus increasingly on effectiveness. Our friends at organizations like J-PAL have been at the forefront of this change, using high-quality evidence to uncover new, highly effective ways to improve the world. Alongside research efforts like the Cochrane Collaboration, which provides systematic evidence about effectiveness, this research enables the work of data-oriented implementers like Evidence Action, an organization scaling evidence-backed programs, to reduce the burdens of poverty. As part of this movement, large institutional funders like USAID and DFID have shifted significantly toward rigorous evaluation and evidence-based policymaking.
We are proud to be part of a decades-long shift toward evidence-based giving. Though our world has tremendous dangers, it also has immense wealth that can be used as a lever to drive improvement, to fill gaps left by the public sector, and to catalyze systemic change. We also have more tools for doing good than any previous generation. We have technological and computational tools, philosophical and conceptual tools, organizational and logistical tools. This post is about those tools — our toolbox for finding the best ways to build a better world.
Differences in charity effectiveness
Scale is only the first of many considerations that we think through in order to help us identify high-impact charities. Our research process is extensive and often time-consuming. But it’s worth a lot of our time, since good charitable opportunities are incredibly rare — and hugely valuable.
One of the most critical concepts behind our work is the idea of differences in charity effectiveness. There are strong reasons to suspect that the best ways to do good are ten, a hundred, or even a thousand times more effective than the average. In practical terms, this means that some charities can save a life for dramatically less money than others. For a given donation amount, you can save several times as many lives by giving to a highly effective charity than by giving to a less effective one.
We conceptualize effectiveness in terms of cost-effectiveness not to be "penny-pinching", but because there are lots of people to help, and only so many resources to help them with. Part of our project is to dramatically expand the amount of money going to effective charities, but at any given moment in time, the amount of money available for charity is finite. We measure cost-effectiveness because we want to know how to make these resources go as far as possible — to help as many people as possible with the resources we have available.
Some good evidence for the idea of dramatically different levels of charity effectiveness comes from the Disease Control Priorities project (DCP2), an ongoing effort that aims to analyze the cost of different interventions designed to save lives and improve health in the developing world.
Here's what the distribution of charity cost-effectiveness looks like for the DCP2 project:
Source: The Moral Imperative toward Cost-Effectiveness in Global Health. DALYs are disability-adjusted life years.
This graph illustrates the reality that the most cost-effective ways to save and improve people’s lives can be more than 20 times more cost-effective than the least. In practical terms, this means that making sure money is spent on the best interventions can save more than twenty times as many lives as it would otherwise. This pattern is repeated in many other domains — for instance, among US social programs and interventions to reduce carbon emissions.
So we keep finding this pattern empirically: a large number of interventions clustered at lower levels of effectiveness, with a “long tail” of dramatically more effective interventions. We have strong reasons to suspect that this pattern generalizes, and therefore a strong motivation to go in search of the charities that work in that long tail.
Why might long tails of effectiveness be common? The reasons for this are somewhat technical, but the following thought experiments will help explain.
Why does cost-effectiveness vary so much?
The first thing to understand is that there is no reason that effectiveness and cost should be strongly related. Loosely speaking, some things are simply more effective than others. Take the (somewhat random) example of the factors you might take into consideration when purchasing a bicycle. A $1000 bike will take you the same distance as a $100 one, but it is ten times less cost-effective per mile traveled. Their prices differ for reasons other than their ability to get you from Point A to Point B: some bikes might be sturdier, more stylish, easier to repair, outfitted with a neat basket, or simply newer. All of these things affect price, but are basically unrelated to how far the bicycle will take you when you pedal it.
With this in mind, imagine that you want to support a charity to help save lives. You identify 1,000 charities that do a thousand different things, all of which help to save lives. Some of those things save 100 lives; some save only one. In fact, imagine that the number of lives saved by each charity is basically random, that is to say, it's distributed evenly across these hundred charities: about as many save 1 life as save 100 lives. This means that if you pick a charity at random from this group, you have as good a chance of finding one that saves twenty lives as you do of finding one that saves eighty lives, or sixty.
Suppose that costs also vary widely across these charities— imagine the work they do costs between $1,000 and $100,000 dollars. The average cost for the work of one of these charities is, of course, about $50,000, and if you pick one at random you're as likely to get a cheap one as an expensive one.
As we've seen with the bicycle example above, cost and effectiveness don't need to be related. Suppose this is also the case with our hypothetical assortment of charities. This is a very realistic assumption: organizations can need more money for reasons unrelated to how effectively they save lives. For example, some geographies are much more expensive to work in and some interventions are much more labor-intensive to deliver.
In order to compare the cost-effectiveness of these hypothetical charities, we want to figure out the number of lives saved for every $1000 spent. The assumptions we’ve made — that cost and effectiveness are unrelated, and that each is fairly evenly distributed — allow us to map the distribution (basically, the histogram) of what cost-effectiveness looks like. This lets us calculate how likely we are to find a charity with a particular level of cost-effectiveness:
We can see in the graph that there are a very small number of charities that are many times more cost-effective than the others. The top 5% — the charities in the 95th percentile — are about three times as cost-effective as the average; the top 1% are forty times as cost-effective; the top 0.1% are about 100 times as cost-effective. This small minority of highly effective charities drags the average level of cost-effectiveness up. The fact that most charities are clustered toward the bottom of the distribution means that if you pick one at random, it is overwhelmingly likely to be worse than average. But there’s an opportunity here as well: if you're carefully choosing where to donate your money, you can literally save 100 times as many lives — as long as you can find the charities in the top 0.1%.
That's what the Founders Pledge Research team does.
Do big differences in effectiveness exist in general?
For many charities that work in global health and development, we can calculate cost-effectiveness by asking for budgets and impact evaluations; with good evidence, we can estimate the direct connection between dollars spent and lives saved. But what about in areas where the evidence is fuzzier, more debatable, or harder to come by? In the past, we've recommended funding organizations with the purpose of reducing the risk of great power war or reducing risks from extreme climate change, where data is sparse and we have significant uncertainties. In these areas, even when we know that the scale of the problem is large, it’s not easy to estimate the effectiveness of charities working on the kinds of interventions that can make a big difference.
We can utilize the same basic premises to identify critically underfunded projects in these areas. Even where there's little hard data available, there are key considerations allowing us to carve up the space of funding possibilities.
Imagine we have 100 hypothetical charities:
Only some of those charities are working on high-priority problems.
Only some of those are transparent enough that we have enough information to evaluate them.
Only some of those have the ability to absorb additional funds.
And only some of the remainder have some previous evidence of effectiveness, which leaves a tiny proportion of the overall number of charities that we should prioritize for further investigation.
In some cause areas, there are specific considerations that point towards greater effectiveness, allowing us to narrow down the space even further. In our climate work, for instance, we think that organizations working on solutions that hedge against the absolute worst climate scenarios are of outsized importance, since the damage in those scenarios is massive. In our work on reducing great power war, we think it's important — but hard — to find opportunities that pose minimal risk of unintended harm. These considerations are the sources of impact differentials — key considerations that divide the highest-impact charities from all others. In our climate work, this involves focusing on neglected technologies and geographies. In our research on nuclear issues, this means working on avoiding escalation, since the most destructive nuclear conflicts would be those that get wildly out of control.
What does the Research team do?
Founders Pledge’s Research team is an internal think tank that studies the world’s most pressing problems and how philanthropists can help solve them. Truly effective giving is hard. If we want to ground our approach to charity in data and evidence, we benefit from understanding meta-science and statistics, using probabilistic reasoning, and developing detailed models of issues ranging from neglected diseases and global poverty to nuclear war and pandemic preparedness.
It also, of course, requires us to investigate charities and implementers themselves. This is the second part of our work: we’re a charity evaluator, assessing the impact of organizations working to solve the world’s biggest problems and recommending those that do so most effectively.
Our work proceeds from a few core ideas:
- We're cause-neutral: our research, our areas of focus, and our grant-making recommendations are driven entirely by impact considerations. We don’t have preferences for certain geographies, topics, interventions, or groups of beneficiaries, except insofar as those concerns are relevant to reducing suffering.
- We’re evidence-driven: We take a curious, critical approach, driving us to understand what is true, rather than what is most convenient to believe. This can lead to conclusions that we don’t initially expect, and cause us to reject beliefs that we held strongly beforehand.
- We care about suffering regardless of how far away it is from us. We care about the suffering of all conscious life, and suffering in the future: we don't care less about suffering if it happens tomorrow instead of today.
Members of the Research team have a wide variety of backgrounds, from science to finance to public policy, but all share one goal: using reason and evidence to find the most effective ways to do good.
Cause selection
Cause prioritization, or choosing which problems to focus on, is one of the most important concepts in our view of charity evaluation. There are some issues that affect hundreds, thousands, or millions of times more people than others. Because we think that all people are equally morally important, we want to try to help as many of them as possible. This is one of the reasons why malaria is a priority cause area: it still kills more than 300,000 children each year — three times as many as cancer, drowning, and car accidents put together. Scale also explains our focus on existential risk: certain kinds of catastrophe could kill huge numbers of people, but the worst could permanently end civilization, turning out the lights not just for us but for our children, grandchildren, and great-grandchildren as well.
As we discuss in greater detail below (“Our research process”), the size of the problem isn’t the only thing that matters. There are problems that affect more people, or that affect people more severely. We think about these problems, too — but we prioritize the ones where we think our work can make the biggest difference. That means thinking, first, about whether solutions exist that can immediately put a dent in the problem and then asking whether these solutions are underfunded.
Cause prioritization can sometimes result in counterintuitive conclusions. It can lead us to helping people further away instead of those closer to home, or addressing obscure or difficult-to-understand issues rather than problems that are visible and visceral. This is precisely why cause prioritization is so important: our emotions are often strong guides to moral behavior, but our brains sometimes fail to give us an adequate understanding of scale. A true accounting of the number of people and animals affected by different global problems often clearly indicates that we should prioritize working on the most severe, largest-scale problems.
Our research process
Step 1: Research prioritization
The first step of our research process is a collaborative prioritization exercise that we conduct twice a year. Throughout the year, we source ideas from trusted researchers, global networks of experts, academic journals, and from organizations and studies that we encounter in the course of our work.
During our biannual prioritization exercise, each team member nominates a handful of ideas that seem especially promising, making educated guesses as to how important the issue is, how plausible it is that we can find a way to address it, and whether or not there are ways philanthropic funding can make a difference. We then discuss, rate, and rank these ideas as a group.
We don’t assign research strictly on the basis of this numerical exercise: in addition to prioritizing potential research topics in this way, we also think carefully about our own comparative advantage as a research organization. Are we the best people to do this research? Could we make a bigger dent by focusing on a different problem?
Crucially, we also allot a portion of our research budget to autonomously directed research; though it’s important to arrive at a consensus about the highest-confidence avenues of research, we think history shows that researchers do some of their best work when they’re out on a limb.
Step 2: Desk research
In most cases, our research process starts with a literature review. When we investigate a topic — say, oral health — a researcher’s first step is to get a comprehensive overview of the area by going through the relevant literature. This step can take anywhere from two days to two months. By the time we’re done, we should:
- Understand the main concepts in the field. In climate change, for instance, this means having a good grasp of the emissions landscape, the main drivers of energy demand, and the broad strokes of the main climate models.
- Have a sense of the key uncertainties and debates. We should understand the main disagreements between experts in the field (would a nuclear war cause extinction?), as well as the key open questions.
- Be able to identify authoritative sources of data — including any flaws. In order to arrive at defensible conclusions when we start investigating our own questions, we need to know which data sources are reliable — and what their main shortcomings are.
- Know who the main experts are — and where their expertise ends. Sometimes, we have specific in-house expertise on a topic, but often, it’s much more efficient for us to ask domain experts than to attempt to dig around in the literature to answer specific questions. But it’s also important for us to understand where expert knowledge is conclusive, and where it’s simply informative.
Step 3: Talk to experts
By the time our literature review is done, we should be bursting with questions and uncertainties. It’s often the case that we aren’t experts in a topic we’re investigating. And experts can usually help us resolve most — but by no means all — of the questions we have. Talking to them is an important step, since we might emerge from our literature reviews with misunderstandings or mistaken levels of confidence.
We seek to engage a variety of expert opinions, map points of agreement and disagreement, and track the origins of the evidence that experts rely on. We spend lots of time trying to understand why experts think the things they do, and following their reasoning to see where it leads.
Step 4: Reason about the evidence
Once we’ve built up expertise, we can tackle the key questions that determine whether we should look for ways to fund work in a given cause area. Those questions are:
- Is this area important?
Some issues affect thousands of people; others affect millions; still others affect billions. All else equal, we want to try to help more people, so our research should help us get a sense of scale. How many lives are affected? How severely? For how long?
- Is this area tractable?
In order for us to commit resources to a cause area, there should be a plausible way for us to make a difference. This doesn’t necessarily mean that there are already granting opportunities that we can donate funds to today. Rather, it means that there should at least be promising ways to make a dent in the problem that are evidence-backed and that a philanthropist could plausibly support.
- Could a philanthropist make a difference?
Some areas, such as air pollution, are dramatically underfunded by the philanthropic sector given their scale. By contrast, there are some cause areas that, in part because they are so incredibly important, receive disproportionate attention and, with it, disproportionate funding. We think that the idea of diminishing returns suggests that donors can make a bigger dent in areas that are less well-funded. This means that we pay particular attention to neglected causes.
In some cases, however, we choose to pursue cause areas that are well-funded because we have reason to believe that existing funding is misallocated. Climate is one such area; although climate change is an area of huge — and justifiable — focus, we believe that it is still possible to find underfunded areas within climate for a philanthropist to make a difference.
Step 5: Look for funding opportunities
Once we’ve characterized a cause area, mapped out potential interventions, and satisfied ourselves that there are ways a philanthropist could add value, it’s time to look for ways to actually donate. Over the course of our research into a cause area, we’ve likely already come across organizations that are candidates for funding. We’ve also built a list of promising interventions. Our priority at this stage is to find organizations that implement promising interventions.
In some areas, only a small portion of the organizations working on the issue are implementing an intervention for which we’ve seen good evidence. For this reason, the set of organizations we consider at this stage has already been winnowed considerably. Still, since we expect to see large differences between charity effectiveness, we keep digging to find the very best giving opportunities.
In order to do that, we need ways to rigorously assess impact. That’s what our toolbox is for.
Our charity evaluation toolbox
There is no step-by-step process for figuring out the best way to create a better world. Doing this work requires asking and answering difficult questions, having hard conversations, and making decisions under conditions of extreme uncertainty. Our team’s work lies in making use of a variety of conceptual tools, which we describe below.
Counterfactual thinking
Evaluating charitable giving opportunities is a matter of figuring out a charity’s effect on the world or, in our parlance, its counterfactual impact. In order to figure out whether some action has an effect, we need to compare two scenarios: the world in which the action was taken, and a “counterfactual” world in which it wasn’t. If there’s no difference between these two scenarios, then we say that the action had no effect. If there is a difference, then that is the counterfactual impact of the action.
The easiest way to understand counterfactuals is in terms of experiments like randomized controlled trials (RCTs). In an RCT, as in a clinical trial, there’s a treatment group, which is treated with some intervention, and a control group, which isn’t. The difference in outcomes between the treatment and control groups is the “counterfactual impact” of the treatment.
We often rely on RCT evidence in our work. Many of the most effective charities we recommend are supported by multiple RCTs, which provide some of the best possible evidence of the impact you can achieve by funding the charity’s work. Against Malaria Foundation is a good example. RCTs have shown again and again that distributing insecticide-treated bednets is one of the most cost-effective ways to save a life.
Without experiments, it’s obviously very difficult to figure out what would have happened in this hypothetical, counterfactual world. But we’re not totally in the dark. The tools that we describe in the rest of this post are, in large part, ways of developing a picture of that counterfactual world.
Expected value
We often confront situations in which we have a lot of uncertainty about which outcome to expect. In these situations, we need a way to decide between different options that are, essentially, gambles. In our work, uncertainty is unavoidable but not unknowable. That is to say, we can’t achieve perfect certainty, but we can put reasonable numbers on our uncertainty.
Imagine two charities:
- For $1,000, Charity A can save two lives with a probability of 50%, five lives with a probability of 40%, and no lives with a probability of 10%.
- For $1,000, Charity B can save ten lives with a probability of 30%, five lives with a probability of 20%, and no lives with a probability of 50%.
Expected value offers a way to think about this problem. The expected value of a gamble, or of an uncertain choice, is essentially a weighted average: the different possible outcomes, weighted by their likelihood. Looking at the problem in this way, Charity B is the better choice by a factor of two:
- The expected value of Charity A is (0.5 x 2) + (0.4 x 5) + (0.1 x 0) = 3
- The expected value of Charity B is (0.3 x 10) + (0.2 x 5) + (0.5 x 0) = 6
How can this be? Can it really be better to forego the virtual certainty of saving at least one life, in the case of Charity A, and risk a 50/50 chance of saving none at all, as with Charity B?
One way of thinking about this is to remember that we evaluate lots of charities. Because uncertainty is unavoidable, we can never be completely sure of making the right decision in any individual case — even in the case of Charity A, there is a 10% chance of saving no lives at all. We therefore need a method for ensuring that we make the right decisions in aggregate, a way to ensure that we help as many people as possible once all of our decisions are taken into account.
Expected value is that method. Under reasonable conditions, the expected value, or expectation, of all of our choices taken together is equal to the sum of expectations of each choice. That’s a mouthful, but it’s a mathematical fact that you can check at the poker table or the roulette wheel: expected value works in real life, which is why we use it in our work.
Cost-effectiveness analysis
Cost-effectiveness analysis is core to our way of evaluating charities that work to save or improve the lives of people living today. Ultimately, our mission boils down to a single, simple goal: to do the most good possible with the resources available.
As we’ve discussed, there are large differences in charity cost-effectiveness, that is to say, large differences in how much charities can actually achieve with an additional donation. These differences make it worthwhile to invest lots of time and effort in cost-effectiveness analysis. When a member comes to us for advice, we want to be able to advise them on how to “shift” their funds from the average charity — which, as we’ve seen, is unlikely to be particularly effective — to one of the most effective ones, so they can maximize the impact of their donations. Because some charities can save ten times as many lives as others for the same amount of money, being able to identify those charities is in itself a life-saving endeavor.
In order to calculate the cost-effectiveness of different charities, we need to do a lot of different things:
- We have to identify how much it will cost to deliver a charitable intervention. This often means looking at budgets closely in order to separate fixed costs (which affect average cost-effectiveness) from variable costs (which affect marginal cost-effectiveness, or the effectiveness of the next, additional dollar). We sometimes also need to forecast cost trends, to see how things might change in future: will the factors affecting costs going forward be the same as those affecting past spending?
- We need to figure out how much good the intervention does. This is often the meatiest part of our work— deriving a concrete estimate for the effect of a given program means combing through academic literature, interpreting impact evaluation data, and sometimes doing a fair bit of data analysis of our own (see this copy of our analysis of Suvita for an example).
- We need to account for uncertainty. Ultimately, our estimates of costs and effects are not certain. As we discussed above about expected value, a best practice here is therefore to calculate expected cost-effectiveness. To do so, we “deflate” our estimates according to our certainty. If a charity seems like it can save two lives per $1,000, but we’re generally only 50% confident in this conclusion1, we deflate our calculation according to this uncertainty. In expectation, then, this charity saves one life per $1,000.
Importantly, our cost-effectiveness analysis work has little to do with how much or how little an organization spends on so-called “overhead.” Metrics like this describe how much of a charity’s income it spends on operational expenses, but they do so without reference to outcomes. A charity can spend almost nothing on overhead and have no impact, or it can spend lots on overhead and have a big impact. The amount of money a charity spends on operational expenses isn’t necessarily related to its actual effects on the world.
Crucially, we don’t only rely on cost-effectiveness analysis. There are some areas where we work — notably, existential risk — where calculating meaningful cost-effectiveness estimates is practically impossible. This is why we talk about having a “toolbox” for charity evaluation; we want to evaluate many possible ways of doing good, in each case using the right tool for the job.
Marginal value
A closely related concept is that of marginal value — the incremental change in output resulting from one additional unit of input. That is, since we advise donors on the best way to spend their money going forward, we don’t necessarily want to know the counterfactual impact of a charity on average. We want to know the counterfactual impact of the next, or “marginal,” dollar.
The marginal value of a donation to a charity can differ a lot, even among charities that do similarly good work on average. This can happen for lots of reasons:
- Charities can have constant returns to scale, in which every dollar spent delivers the same value.
In practice, we think this is unlikely to be true at a large scale — most charities could not absorb a trillion dollars, for instance, without becoming less effective. Still, it can be approximately true at a smaller scale.
Many of our highest-rated charities, like Against Malaria Foundation, have low fixed costs and a massive population of potential beneficiaries. This means that additional funds simply allow them to serve more beneficiaries at roughly the same level of cost-effectiveness.
- It’s often the case that charities have diminishing marginal returns, suggesting that the value of a marginal dollar is lower than the value of previous spending.
One way of thinking about this phenomenon is that it’s about picking the low-hanging fruit: we might assume that this is the case if charities are likely to pick the highest-value activities to work on first, which means that additional donations finance lower-value (though by no means low-value) activities. Often, charities simply can’t spend money as fast as they receive it, and it’s also possible for them to saturate the geographies or where they work: the closer they come to such a point, the lower the impact of the next dollar they receive.
We sometimes recommend that donors restrict their grants to certain programs, even when the organizations that host these programs could absorb much more funding. We do this when we think that the program we’ve highlighted is dramatically more effective than others at the same organization; in other words, when marginal returns drop off steeply.
- If there are returns to scale, the value of an additional dollar can be higher than the value of the average dollar.
This might happen if, for instance, additional funding “unlocks” new projects that are higher-value than those that have come before. Some of the best catalytic opportunities we uncover look like this: using new funds to expand an existing program to a region where it can be more cost-effective is an example of increasing marginal returns, because a dollar spent on the expanded program will achieve more than a dollar donated before the expansion. The Founders Pledge Climate Change Fund’s work catalyzing the expansion of the Clean Air Task Force is one such case.
- The value of a marginal dollar can also be zero.
This can happen when charities get saturated with funding, and simply have no capacity to use an additional dollar. We think this situation is a lot more common than many donors might imagine, particularly for some of the highest-profile charities that attract many millions in annual funding.
This means we tend not to recommend charities that are unusually good at fundraising, or that receive a lot of public attention or support. Though it’s not always the case, these charities are often sitting on more money than they can use, and donors would be better off giving to a charity that can use the funds to help someone in need immediately.
Bayesian reasoning
It’s typical to think of the world in terms of black and white: a hypothesis is right or wrong, an intervention works or it doesn’t, a charity is good or bad. We don’t think in those terms. Instead, we think in terms of degrees of belief. We don’t ask whether an event will happen or not — instead, we ask what the probability is of that event happening. Moreover, we try to incorporate prior knowledge so that we don’t need to pull probabilities out of thin air.
In contrast to frequentist inference, which treats probabilities as facts about the world (“there is a 50% chance of rain tomorrow”), Bayesian inference views probabilities fundamentally as statements of belief that can be adjusted according to new evidence (“I am 50% confident that it will rain tomorrow”). Thinking of probabilities not as fixed but as fluid representations of our confidence allows us to update them in response to new evidence. This is a good thing.
In Bayesian inference, we typically have a prior belief and some evidence. We combine those to form a posterior — an estimate of the probability of an event that takes into account new data in light of prior knowledge. This process is known as “updating.”
Why be Bayesian? One important motivator is efficiency. Our task is to do the most good we can in the world with limited resources. In charity evaluation, information is one such limited resource: there is so much we don’t know. That means that we need to make the most of the information that we do have, relying on all of the available data, including indirect evidence, to try to triangulate the truth.
We use Bayesian inference in a number of different ways:
- We use scientific background evidence to propel our reasoning about different interventions. Much of our evaluation of the Lead Exposure Elimination Project, for instance, is based on prior scientific evidence about the severity and consequences of childhood lead poisoning.
- We sometimes do explicit Bayesian updates on data using statistical programming languages like R and Stan. This has been useful, for instance, in our assessment2 of StrongMinds and in a grant our Global Health and Development Fund made to r.i.c.e. Institute. In each case, we had prior evidence about the intervention implemented by each organization, and used charity-specific data to update that prior evidence in developing a final estimate of impact.
- More informally, our team tries to maintain a Bayesian culture. In practice, this means that we try to build norms and processes around responding actively to new evidence, quantifying our intuitions, and incorporating appropriate prior evidence.
Meta-science
One of the most challenging parts of our work is the need to interpret evidence from a wide variety of different sources. How should we think about the evidence? Should we find the experts and do whatever they tell us? Should we start from first principles and draw our own conclusions? What about academic papers? Should we take them at face value? What do we do when two pieces of research disagree?
Meta-science, or the study of science, is one of the disciplines most fundamental to our work. We don’t typically run randomized controlled trials (RCTs) ourselves, and only on occasion do we analyze beneficiary-level microdata about specific programs. We often rely on academic papers and other published research to try to answer key questions that bear on our decision-making.
But we can’t simply look at a paper and trust its results. For one thing, there are many topics where experts vehemently disagree. Sometimes, we need to dig in simply to understand the substance of the disagreement, which can be esoteric. Moreover, the quality and reliability of published research varies dramatically.
John Ioaniddis’ seminal 2005 paper, Why Most Published Research Findings Are False, argued that, because of the way hypothesis testing influences what papers are published, a high percentage of published papers are simply false. Though these findings may have been overstated, Ioannidis’ paper brought attention to the way that structural and methodological factors in research and academia can generate conflicting, uncertain, and downright wrong results.
The ongoing replication crisis has validated these concerns: in many disciplines, major findings simply cannot be reproduced when researchers rerun key experiments or take a second look at the data, suggesting that the claimed effect may have never existed at all. Some of the most highly publicized findings have suffered this fate: power posing, to give one example, was the subject of a prominent TED Talk before the original study’s results were cast into doubt.
It turns out that there are lots of things that can go wrong during the research process:
- “P-hacking” is the practice — not necessarily nefarious — of trying different combinations of statistical analyses until a statistically significant result emerges. Such results can and do appear by random chance, but it’s not yet common practice to report the null results from the analyses that didn’t turn up a significant result. This means that there can end up being an over-representation of positive results (relative to whether there is a true effect or not) in the published literature.
- Publication bias is the existence of scientific literature on a topic that represents only one portion of the available evidence, typically the evidence that shows a large effect or an impressive correlation, while studies that show no effect are never published.
- Underpowered studies in disciplines such as political science are those that don’t have a large enough sample to detect real effects and for which, dangerously, effects that appear to be real are more likely to be illusory.
- It’s also common for research to mistake correlation for causation, often using causal or pseudo-causal language: a putative cause is “linked to” some effect, when in reality the two variables are simply related by some hidden third factor. This is a particularly important concern for us, since we often want to figure out the effects of an intervention (immunization incentives, cash transfers, etc.) that a charity is implementing. It’s crucial that we know the intervention causes an outcome, instead of simply being related to one. This is where RCTs can help.
- Implausible generalization occurs when a research paper makes a claim that is bigger than what’s justified by its actual findings. Though this is a common issue in psychology, it’s something we need to watch out for more generally: we don’t want to draw unwarranted conclusions from narrow studies. A good example of implausible generalization is the claim that “hot drinks help you cool down”: this may or may not be true, but the study on which the popular claim is based consisted of nine men on exercise bikes in a laboratory.
There is no one way to deal with all these issues. As a research team, we need to constantly improve our level of methodological sophistication in order to be able to understand, interpret, and critique research. But we also need to use heuristics to improve our efficiency: we can’t spend all of our time replicating studies.
The credibility revolution that started in economics in the 1980s is key for us. The use of randomized controlled trials (RCTs) to estimate the effects of international development interventions means that we now have good-quality causal evidence to work from. When we’re trying to figure out whether an intervention works, RCTs are the gold standard. The charities we most confidently recommend are often backed by this kind of evidence, which allows us to draw much more reliable conclusions than observational studies do.
We don’t always have RCTs to rely on, but there are other types of research design that allow us to have more confidence in results than we otherwise would. So-called quasi-experimental designs3, if done well, can provide evidence almost as good as we can get from an RCT by finding ways to cleverly simulate the random assignment that occurs in an experiment.
But our best tool for evaluating scientific research is, ultimately, our skepticism. We don’t think it’s good to be reflexively skeptical about new findings, but we try to approach our work with a Scout mindset — we want to go wherever the evidence leads us, while being very careful not to be led astray.
Judgmental forecasting
Since we’re in the business of recommending charities for donations, and since we’re interested in the marginal value of those donations, our work depends, to a certain extent, on predicting the future. In many cases, we need to be able to incorporate those predictions directly into our cost-effectiveness analyses. That means we need to put numbers on our predictions. That, in turn, means that we can check and keep score on our predictions after the fact.
Instead of saying we think something is “extremely likely to happen” or “somewhat unlikely to happen”, we say that it has a 95% chance of happening, or a 40% chance of happening. After we’ve made and assessed enough forecasts, we can check our calibration: we say we are “well-calibrated” if things we say we have 50% confidence in happening end up occurring about half the time, and if things we say we are 99% confident about happen nearly always.
This means that we make internal forecasts about all of our charity recommendations: everything from how much money they’ll raise in the following year to the results of impact evaluations.
It’s not the case that “you just can’t put a number on it”: if you’re ignorant about a topic, this should increase your uncertainty, and uncertainty is quantifiable. Being well-calibrated is a matter of accurately estimating our own ignorance. As the work of Philip Tetlock and Barbara Mellers shows, well-calibrated amateurs can outperform even highly trained experts when forecasting world events. This line of research has led to valuable insights that we use in our own research.
A good example of the importance of forecasting in our work appears when we evaluate or recommend charities that advocate for policy change, such as Clean Air Task Force. In these cases, we need to know how likely they are to succeed in order to calculate the expected value of a grant to them. Whether a grant is worth recommending in such a scenario hinges on how likely they are to succeed in this endeavor. Is their chance of success 1% or 0.001%? Accurately distinguishing these probabilities makes a difference of a factor of 1000 in the ultimate expected value of a grant. This is why getting calibrated is vital.
Rhetorical reasoning
Ultimately, not everything is easily quantifiable, and we want to be wary of evaluating only things that are amenable to some kind of mathematical analysis. Though we try to put numbers on things as much as possible, there are some areas where it can be more fruitful to attempt to reason through our recommendations in a different way. We sometimes think of this as “legal-style” reasoning: in these types of evaluations, a researcher “builds a case” for a specific recommendation, while other researchers poke holes in it.
In our work on Great Power Conflict, for instance, the kinds of interventions we study — track II dialogues, research on de-escalation measures — are the first step in a long causal chain leading, hopefully, to reduction in catastrophic and existential risk. In order for a grant to an organization in this space to be a good idea, lots of different things need to be true.
We evaluate these key considerations one by one, building the best case for recommending a given grant. Then we tear it apart. Our research process makes copious use of red teaming, in which internal and external reviewers evaluate research products with the goal of poking holes in them. Only grants for which we have watertight cases are recommended.
Impact multipliers
Our research into specific causes helps us understand which factors influence effectiveness within a cause area. The use of these heuristics enables us to rapidly distinguish the organizations and initiatives that are most likely to be effective from those that are probably less effective.
To give a simple example, if some interventions within a given cause area are several times as effective as others, then we should prioritize charities that implement those interventions. If a problem is worse in some regions than in others, we should prioritize charities working in those geographies.
A key advantage of impact multipliers is that they enable us to answer what are essentially yes-or-no questions about charities working in a given cause area. Are they working in Geography X? Are they implementing Intervention Y?
To develop these multipliers, however, we need to conduct extensive research. A good example of research that was used to develop impact multipliers is our report on air pollution, which guides our grant recommendations and direct grant-making in that area. Our research on that topic informs our search for effective organizations working on air pollution: among other multipliers, we look for organizations that work in the areas where pollution is worst, where it’s currently getting worse, and where the sources of air pollution are most affectable. Having these impact multipliers to hand drastically reduces the size of the search space we need to look at to find effective charities.
Impact multipliers are particularly important when we consider charities, interventions, and cause areas for which cost-effectiveness analysis is impractical. When prioritizing charities that work, for instance, on preventing great power conflict, we have very few usable numbers to work from. What we do have is a research-based sense of what types of activities and focus areas are most likely to make a dent in the problem.
Continuous iteration
In the long run, the best way for us to maximize the good we do in the world is to have a process that generates good outcomes. The set of tools we’ve described here characterizes the current state of that process, but we can always improve. That’s why the final step of our research process is the most important part of the work of our research team: taking the toolbox we’ve just described, and throwing out its contents, piece by piece, in favor of better and stronger tools. The most important part of our research process is iteration. We are proud of our toolbox, but it’s always changing.
If you have an idea for how we can do things better, or if you want to share thoughtful critiques of any of our research or recommendations, we hope you’ll reach out to us at research@founderspledge.com.
Notes
-
There are a number of factors that affect our confidence levels. One of the most common deflators is an “analysis quality discount”, where we try to account for the possibility that our own analysis might itself be in error. We also try to account for things like geopolitical risk, organization-level considerations, and our confidence in the quality of the evidence surrounding a given intervention. ↩
-
You can look at our Bayesian analysis for StrongMinds on GitHub. ↩
-
Quasi-experimental designs, though not actually experiments, offer some of the same advantages as RCTs: by making use of “natural experiments” and other types of random variation in the world, these types of study can help analysts come to conclusions similar to those that a well-designed RCT would facilitate. ↩